Financial institutions are no strangers to AI-powered automation. From delivering security codes and handling customer transfers to closing and blocking accounts, AI automation in financial services comes with a plateful of efficiency-boosting use cases.
And, while most use cases in this sector revolve around structured, tabular data (think algorithmic trading or risk management), it's the day-to-day, unstructured data that remains underutilized in the industry.
Automating financial processes often involves human decision-makers for portfolio optimization, fraud detection, and sales/marketing optimization.
Admittedly, structuring and studying copious amounts of data is a tedious task, making data abundance a curse. The best guess is that you're on this page to figure out how to help organize and analyze data while automating processes for better productivity, happier employees, sector innovation, and reduced operational costs.
In this article, we’ll highlight the role of AI in financial institutions, and show you some real-life examples of how to get started - even if you don't have your processes all figured out yet.
AI in financial services: How to deal with data overload?
The approaches to using automation tools differ greatly based on what type of financial organization you're working in. But: No matter if you're going for Robotic Process Automation for back-office tasks, or are looking to streamline your processes with structured data analysis, there is always one thing at the center of your strategy: the customer.
And customers - their interactions, communication, and management doesn't always fit int the nice, neat Excel sheet. Let's face it, most organizations put a lot of focus on structured data analysis, and qualitative data is often overlooked or underutilized.
And that's for a very simple reason - it's very, very difficult to extract meaningful insights at scale. That's where AI comes in.
Machine Learning & Natural Language Processing tools can help you sift through these data in real-time - as long as you know what parameters matter to you, there is a way to get to results.
A word of caution, however: there is no tool that can do it all. Establishing the groundwork for your automation strategy also means mapping out your most crucial steps sequentially. There are two schools of thought on this matter:
- Start with structured data analysis, and enrich these data with unstructured data analysis.
- Automate your unstructured processes in parallel to tabular data and combine these from the get-go.
While both approaches are plausible, we tend to lean on the latter for a few reasons:
- Uncovering previously unrealized opportunities in real-time.
- Improving customer experience and uncovering what makes them return.
- Reducing operating errors - and cutting back the time going into task handling and marketing new services and products.
Whichever automation approach you're going for, evidence shows that the winning strategies highlight the need for a holistic AI implementation that extends across business lines, usable data, and qualified employees, and put the customer in the center.
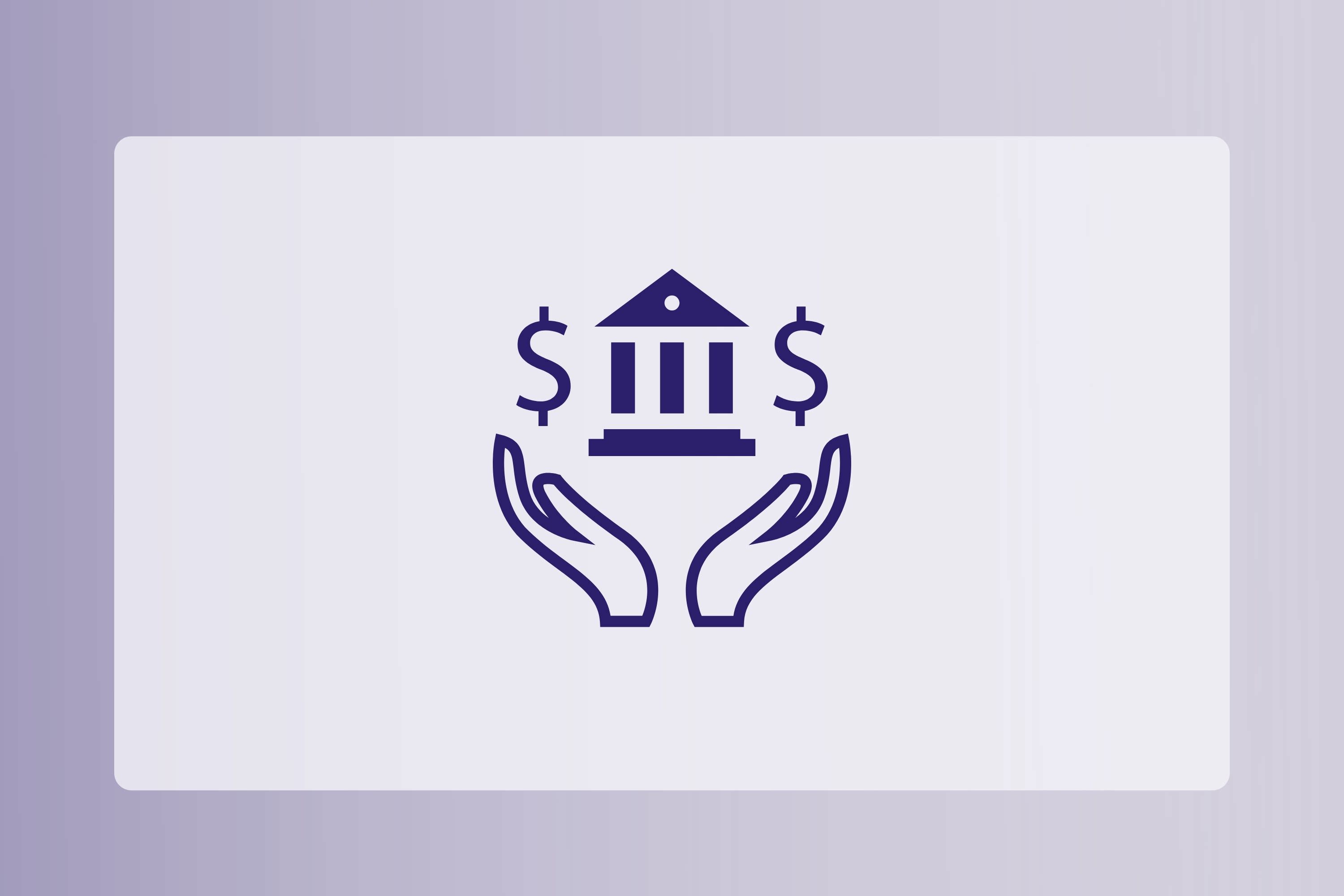
AI in Finance: Use cases & best practices
Despite the process challenges, 80% of finance leaders have implemented or plan to implement RPA. However, only 15% have been able to scale AI.
Even so, the future for AI automation in financial services is bright. Moving forward, two things, in particular, can make implementing and scaling AI easy:
- Combining different technologies to automate complex processes.
- Using the low-code tech that doesn’t require coding and programming skills.
Let's dive into some use cases!
AI & Risk Management
It’s never easy – even humanly possible – to analyze large amounts of data quickly without automation. AI can manage all data including unstructured data at a fast pace - minus the odds of error.
As a result, the financial sector can use AI to analyze data showing the history of risk cases to identify symptoms of potential issues before they grow.
AI can also monitor real-time activities in a specified environment and market. Consequently, it helps make accurate projections, and text generation tools can create reports with detailed forecasts based on several variables – assisting in growth planning and more.
While main use cases in this area are all about utilizing structured data - where unstructured data makes a difference, is in risk monitoring. Once you've understood the parameters that affect your base, you can fine-tune a Machine Learning model to identify and pick up any of the multiple variables - and think about a problem like you would.
AI & Fraud Prevention
Laborious manual processes involved in monitoring money laundering make fighting crime an inefficient and costly process. Money laundering contributes to a 2-5% loss to the global GDP. In 2020 alone, banks paid $10.4bn in fines for money-laundering violations.
But - real-time automation can help. By collecting customer and counterpart data from various sources (think internal sources and external sites), AI can help build strong AML processes.
Combined with unstructured data, that focuses on customer behavior, verifying documents or identification, and checking the completeness of files, you can build a system that identifies the right variables to keep an eye out for.
AI and Trading
Algorithm trading uses automated technology to analyze large data volumes for executing trades quickly and effectively. And while these algorithms are working on large amounts of historic tabular data, there are automation tools that can help you get started (albeit, Levity not being one of them)

Essentially, these models focus on two things: making quick and accurate trade decisions based on market evaluations and identifying trends and patterns in market price changes. The chief aim of algorithmic trading is to assist in maximizing market returns for your investment funds. In doing so, it helps to reduce the cost of manual work that goes into processing trades.
AI and Process Automation
AI’s use in process automation is extensive. Operational costs and employees focusing on mundane, day-to-day tasks culminate in client experience taking the backseat. No wonder, customers who say they’re sure of leaving their bank blame poor customer service as their primary reason. 56% of those who have left say the bank could have changed their mind.
Simply using AI-powered character recognition can automate many of the extensively manual, time-consuming tasks that occupy several work hours.
Similarly, using finance reporting automation with AI-enabled software, you can get help with data verification and report generation. From there, AI takes over data sorting or extraction from forms and agreements, document reviews, and more.
Speaking of specific use cases, let’s look at wealth managers. They can leverage automation to reduce manual work involved in setting up client accounts, identification and authentication of customer profiles & deepen customer relationships.
.png)
Traditionally, this process is packed with a lot of paperwork and tons of exchanges between wealth managers and clients. However, streamlining the process using a combined trio of digital forms, automation, and Optical Character Recognition (OCR) helps:
- Dial down the set-up time.
- Reduce room for human error.
- Drastically improve customer experience.
AI and Document Management & Compliance
About one-third of community banks spend upwards of 5% on compliance. According to the same report, only 1.8% expect their compliance costs to decline by at least 5% - and 15.9% say compliance regulation is among the greatest challenges that banks faced in 2022.
Such rising operational expenses paired with increasing regulatory requirements impact banks’ performance.
The list of documents that reps, service, and support should manage in the financial sector are... well, countless. Here are a few examples of AI Automation:
- Checking documents for missing input and completeness - are all the signatures present? Are there any missing fields that should be filled?
- Helping insurance providers identify fraudulent claims and providing relevant insurance policy suggestions to customers.
- Managing client onboarding documents at scale and making sure everything ends up in the right place.
- Building workflows to manage incoming documents correctly.
- Work through legal and regulatory documents.
But compliance doesn't only affect documents. You can also set specific rules for how incoming communication is managed.
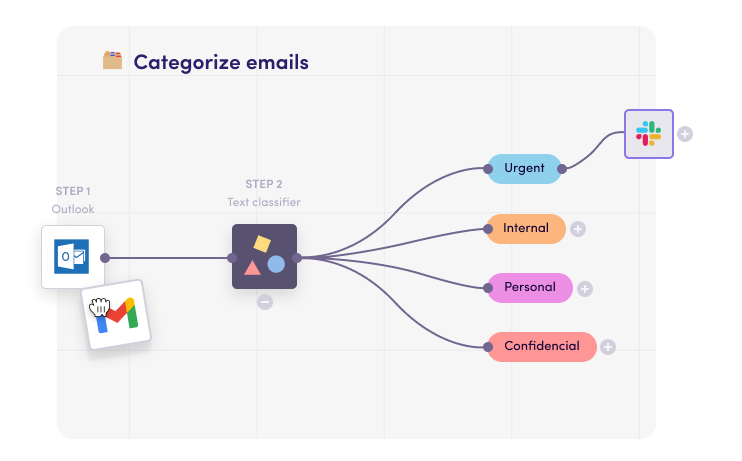
By embracing digital workflows, any organization can take a process-driven approach. This, in turn, can help them understand their target audience better and create new customer satisfaction-boosting digital services.
AI and Investment Analysis
Financial services can use NLP to study the competitive landscape and evaluate investors.
By evaluating environmental, social, and governance (ESG) ratings, investors can determine who to invest in. In that case, a high ESG rating is a telltale sign of better profitability for an investor.
On the other hand, firms looking for investors can use AI to their benefit too. Together with NLP and sentiment analysis, they can compute their own and their competitors’ ESG ratings. This helps them understand their organization’s standing and their status as compared to others.
Now, if you're a smaller firm, your process probably involves looking through company descriptions on platforms like LinkedIn or Crunchbase. Even after rule-based filtering, you'll likely have to manually read through the descriptions.
.png)
You could alternatively train an AI model to think like you do based on historic decision-making on company descriptions, pitch decks, or any other unstructured, qualitative data you're working with.
Why financial services need AI automation
The financial services sector showcases two ripe reasons that set the stage for finance automation:
- One, the industry grapples with mounds of unstructured data. This data needs to be unlocked, managed, and leveraged for efficiency and better decision-making at scale.
- Two, the data needs to be presented to stakeholders and clients. Again, organized data here will help automate customer needs effectively.
With AI, the financial sector can structure and use data consistently, accurately, and efficiently.
And while the balance of tools out there is skewed towards structured data, we'd be happy to chat about your unstructured processes - and how to build a workflow that encompasses both.
If you're ready to get started, we'd love to hear from you!