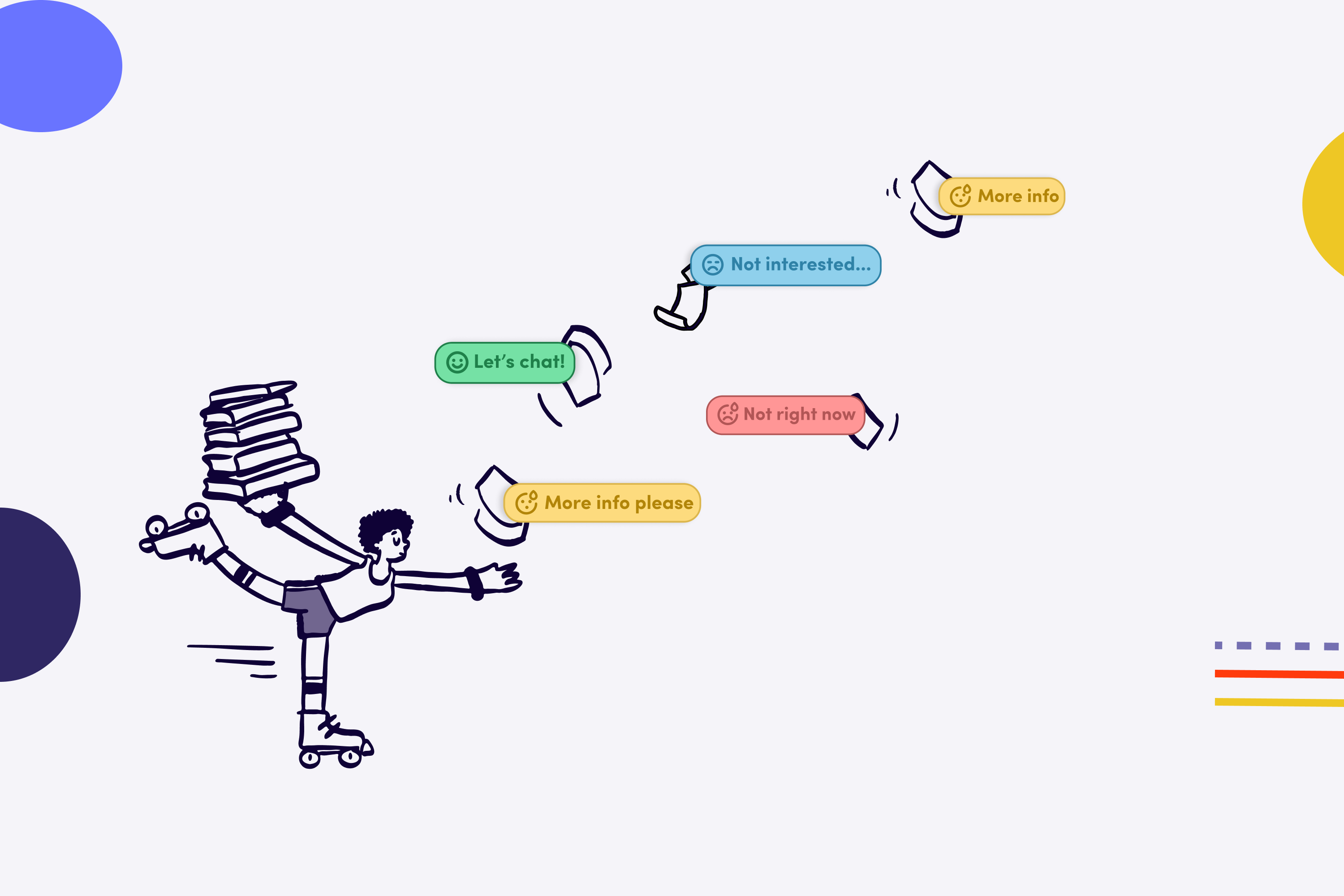
AI for Customer Support and Why You Need It
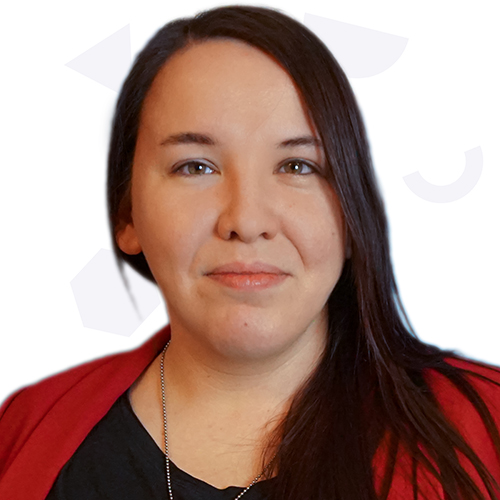
Hanna Kleinings
Customer Operations Manager
The growth of Artificial Intelligence (AI) is setting the stage for increased efficiency across companies, especially when it comes to customer service.
AI-powered customer support enables you to develop deeper insights and build a better user experience. This leads to improving online customer experience, retention rates, brand image, preventive help, and even the generation of revenue. In fact, AI is expected to boost company productivity by up to 40% by 2035.
Although chatbots are a popular approach to AI in customer service, modern AI solutions offer much more. Customers and customer service professionals unlock a new perspective with technologies like Machine Learning and Natural Language Processing (NLP).
How do they do this?
We’re glad you asked—read on for more on AI customer support software and its uses.
How can AI automate customer support?
Customer service is a vital consideration for 96% of consumers across the globe when it comes to deciding whether or not to stay loyal to a business.
Artificial intelligence is the key to enabling real-time service for customer support platforms. What’s more, this technology has the potential to shift the way customer service solutions are developed.
First, we’ll take a look at how AI works, and then we’ll discuss the different ways you can use it to automate customer service tasks.
How does AI generally work?
Artificial Intelligence is a general classification that refers to a variety of technologies—-from Machine Learning to Computer Vision.
Put simply, AI allows you to create and train a model. You begin with a certain amount of data, structured or unstructured, and then teach the machine to understand it by importing and labeling this data.
When it comes to Artificial Intelligence in customer service, we’re typically talking about natural language processing (NLP)—a subset of Machine Learning.
Machine Learning enables computers to perform a task without being explicitly programmed to do so. It instead uses algorithms to perform specific actions by recognizing patterns in previous data to make predictions with new data.
It’s an AI segment that can process vast amounts of data and quickly extract insights. The customer service professional first establishes the rules and then the Machine Learning model does the rest.
For example, customers inquire and support staff respond to those queries which create enormous volumes of decently organized data in customer service. Machine Learning helps a program collect and process this data, and train itself to understand and respond to client requests. Often, this necessitates the use of extra technology, such as NLP software.
NLP enables machines to understand spoken and written messages. For example, chatbots and assistants like Siri and Alexa use NLP to interpret what the user says and provide a response.
AI in customer support generally uses these two approaches to assist both users and customer service representatives. The way we use AI models for customer support often depends on whether we’re working with structured or unstructured data—or maybe even semi-structured data.
Structured vs. unstructured data
Data analytics software can easily examine structured data since it is quantitative and well-organized. It's data that has been organized uniformly—which enables the model to understand it.
Structured data examples include NPS scores, analytics information, and close-ended questionnaires.
Unstructured data lacks a logical structure and does not fit into a predetermined framework. Audio, video, photos, and all types of text—such as responses to open-ended questions and online reviews—are examples of unstructured data.
Semi-structured data, which has a flexible organizing principle, is in the middle of these two categories of data. For example, messages from customers on your CRM tool can be structured according to the process or feature they refer to, but the content of the message is still unstructured.
Your AI model is only as good as the data you feed it—knowing how you can use your data is the key to uncovering AI-powered insights. Let’s take a look at some real examples of how you can use automation tools in customer service.
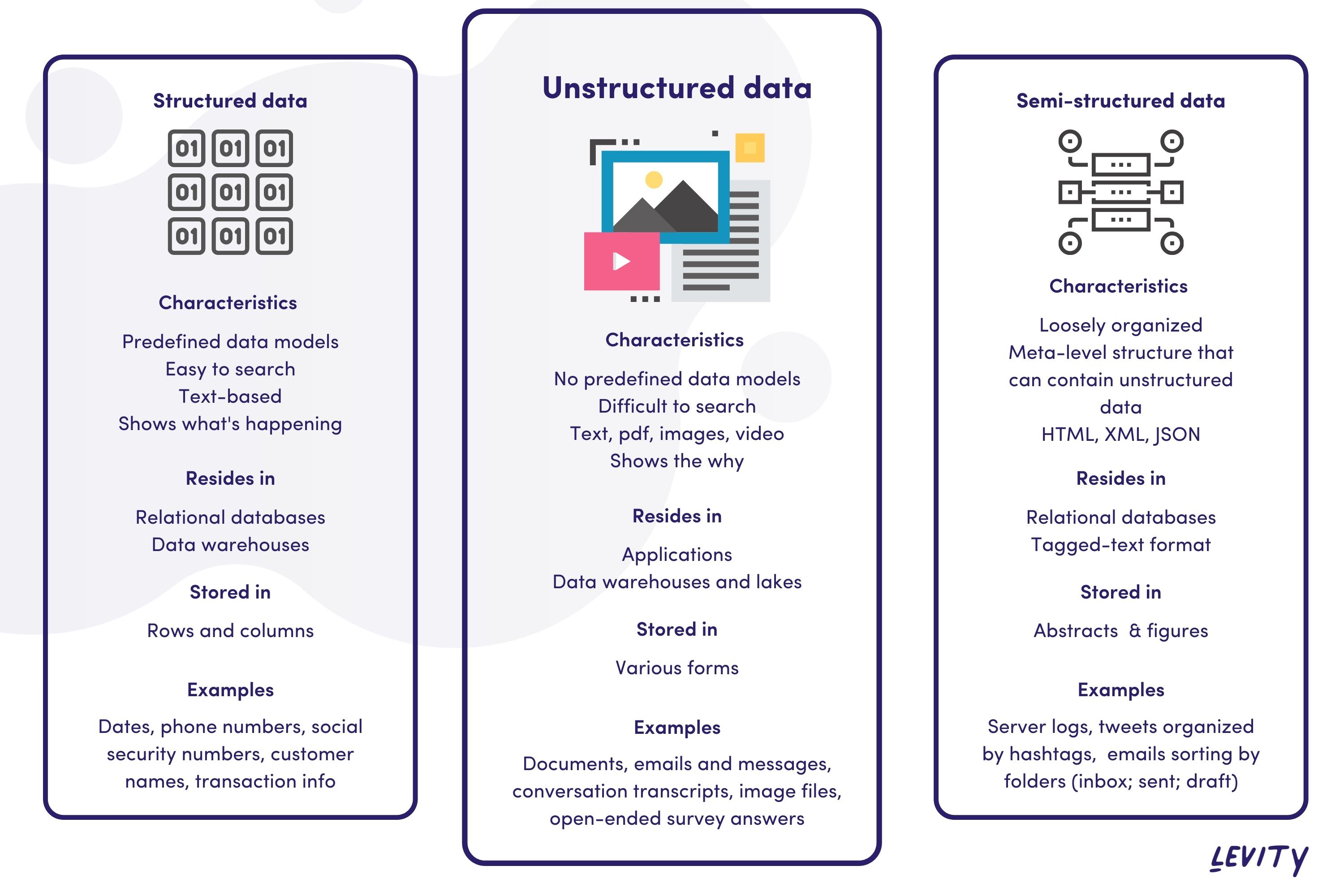
Use AI to categorize support tickets
Thanks to automatic tagging tools, you can easily categorize customer support tickets. This means that you add labels to your data that help you structure it so you can process it easily. You can choose to tag your tickets according to various topics.
For example, you could tag your tickets according to the feature they relate to. Each ticket is analyzed and categorized as relating to a specific feature, and your team has a better idea of what’s causing issues among your users.
.png)
Note: With Levity, you can then instruct your model to notify the specialized support specialists in charge of managing support for those features.
Use AI to perform Sentiment Analysis on customer surveys and feedback
If you have open-ended responses in your customer surveys, you can analyze their sentiment. The most straight-forward categorization you can do here is to see if a response is:
- positive
- negative
With Sentiment Analysis, you can find out which components of the customer experience have the biggest emotional effect.
For example, AI-powered Sentiment Analysis of a customer survey could uncover that users are ‘dissatisfied’ with one of your core features. This enables you to prioritize the development of this feature based on the feedback you’ve received.
Note: For more on how to make customer survey feedback actionable, check out this article.
Use AI to analyze text
Just like analyzing the sentiment of tickets, you can also analyze pieces of text—such as customer support queries and competitor reviews. You just need to set up the tags you want the AI model to use when analyzing and categorizing your text—as demonstrated below.
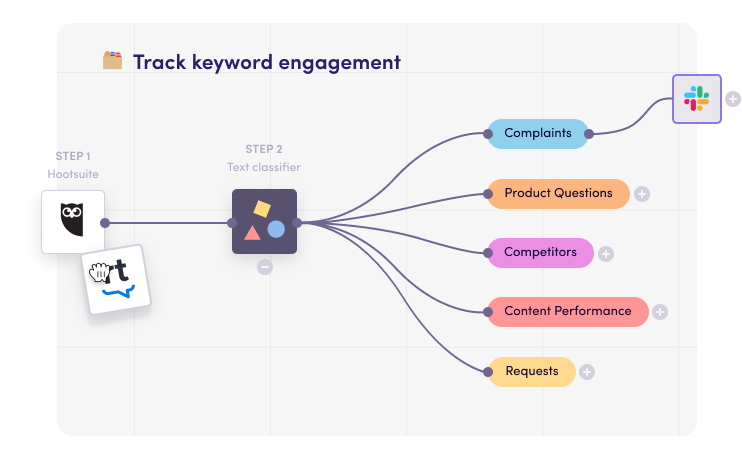
Regardless of the data format or name, automation technologies can recognize the underlying mood, purpose, and urgency of bodies of text. The AI model examines the content and applies one of the tags you've trained your model to recognize.
Use an AI customer service chatbot
Many businesses currently employ chatbots to answer basic queries using information gathered from internal systems. This includes things like delivery dates, owed balances, order status, and more.
By creating an AI-powered chatbot to answer frequently asked questions with customer-specific information, your customers will be able to get answers to their questions more quickly and simply. In turn, this enables the customer support staff to focus on more complex issues and provide a better overall experience while lowering operating expenses.
Use AI to provide multilingual support
With automation tools, you can detect languages and provide a response in your user's preferred language.
When you have an international product, multilingual customer care can help you attract and retain clients. You can transform them into ardent brand supporters by assisting them in getting higher benefits from your products or services in a language that suits them.
Use AI for sorting & routing incoming emails
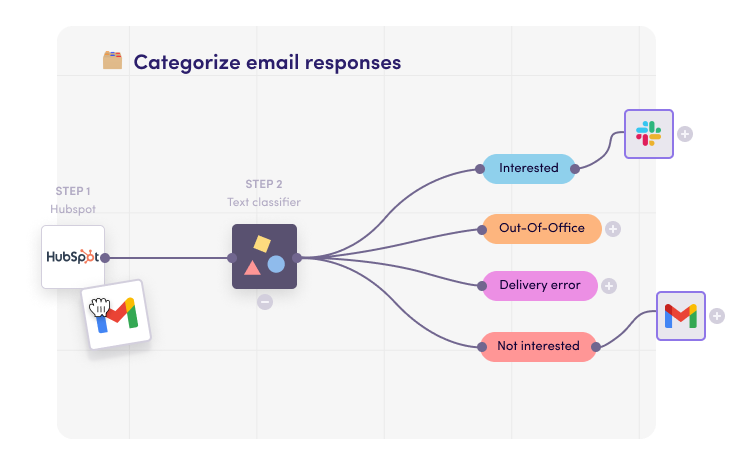
Use an AI-powered tool to automate email sorting into different actionable datasets. You can opt to respond manually, automatically, or be alerted of urgent requests based on the tag.
For example, if the email response is categorized as Out-Of-Office, you can send another reminder to this prospect after a week.
You may also receive specific insights on the performance of your campaign by aggregating the categorized answers in one place. You can then run analytics on your data to uncover greater details by integrating your model with other solutions.
What are the benefits for the customer?
Let’s see how the customer experience improves when you implement an AI tool in your customer support process.
Super-fast support
AI enables you to set up automated responses to customer requests—meaning instant replies where possible. Trickier problems are streamlined to the relevant support agent’s inbox, and they’re able to provide solutions and support faster than ever.
This makes problem-solving much faster and improves the overall customer experience.
Guaranteed consistent support
Even if there are no available representatives at the moment, automation tools allow you to provide consistent support. Your customers will be able to solve a problem at any time of the day with AI-powered customer service bots.
Reduction of human error
We all make mistakes—but AI-based models are trained to be accurate and precise. The more data they process, the more accurate they become. This means that the more you use it, the better results you will get.
Greater accuracy will ensure that you stay on top of evolving customer support needs. Eventually, you’ll create a better experience for your customers.
What are the benefits for the company's customer support?
Now, let’s take a look at the benefits of AI-powered customer support for your organization.
Lead identification
Automated customer service processes can help you identify potential leads that could turn into customers. Once a contact enters your system, you can get them into marketing flows that guide them through the customer journey.
For example, if you’ve sent someone a welcome email with a Call to Action, you’re probably tracking whether they’ve clicked or not. With automated marketing flows, people who didn’t click could get an automated reminder a week later. This can help you increase conversion rates and revenues.
Collecting information
AI enables you to collect large amounts of information quickly and effortlessly. You can turn this information into actionable steps that improve your product and your customer service process.
For example, if you have automated text analysis, you can process a number of customer messages. When you see a certain word or phrase keep repeating, this could mean that there’s a constant problem with a particular aspect of your product.
This could help you notice trends and make product changes that will eliminate the problems customers are facing.
Increased brand reputation
Having satisfied clients means having brand advocates who will promote your brand far and wide.
If you have a large number of customer messages and you’re processing them all manually, you might not be able to get to them all. This isn’t the case if the process is automated—you’ll be able to get to all of them.
Customers are happier when they get speedy support, and happy customers are stronger brand advocates.
How to get started with AI for customer service
Getting started with customer service automation is a straightforward process when you’ve got the right tools. Let’s see how you can do it with Levity.
Upload the data you’ll use to teach your AI model
The first step is to add your data to the system. Your data could be:
- Internal: from a file you already have, such as a Google Sheet
- External: from other integrated apps, such as your CRM tool
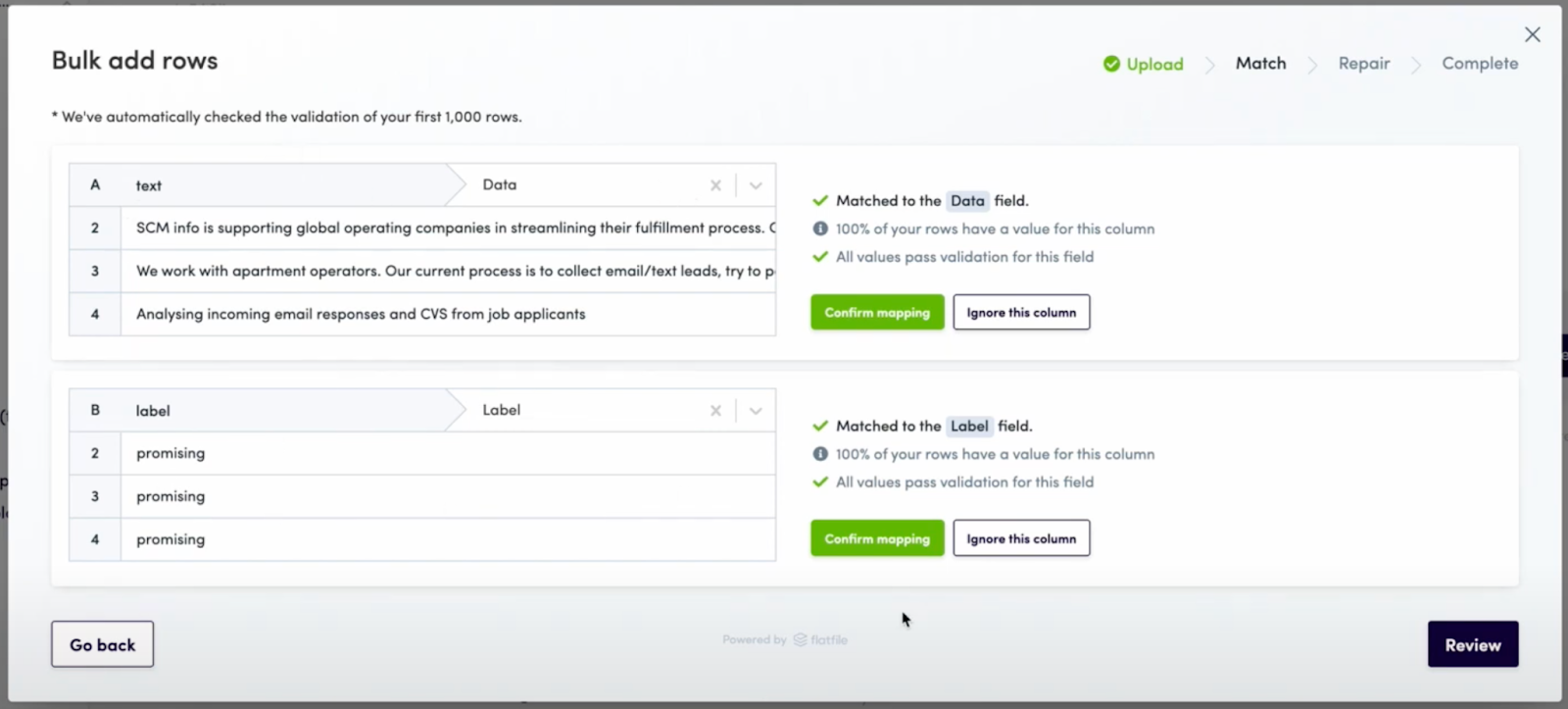
Design your labels for categorizing your data
The content should match the labels you've specified. That's how you'll train your own AI model to categorize data according to your specifications.
These labels give meaningful information for the algorithm to utilize as a benchmark, which includes the input data points and the final outcome you're looking for in your model.
For example, let’s consider some labels for a number of different use cases for AI in customer service:
- Categorize support tickets: billing, feedback, question, complaint.
- Categorize emails by content: urgent, internal, personal, confidential.
- Sentiment Analysis: positive, neutral, negative.
- Classify email attachments: invoice, CV, quote, contract.
Your labels depend on your data and what you’re looking to identify—once you’ve ascertained this, it’s time to train your model.
Train a model
The process of training your data involves uploading data—whether that’s text or images—to one of your predetermined labels. This data is called ‘training data’, and it essentially gives the AI examples to learn from. You can use internal data—your own data, or external data—data taken from other sources.
You want to include at least two labels and a minimum of 20 data points to your model to effectively train it to produce more accurate results. The machine will improve in precision and accuracy over time—the more data you provide, the more precise the predictions will be.
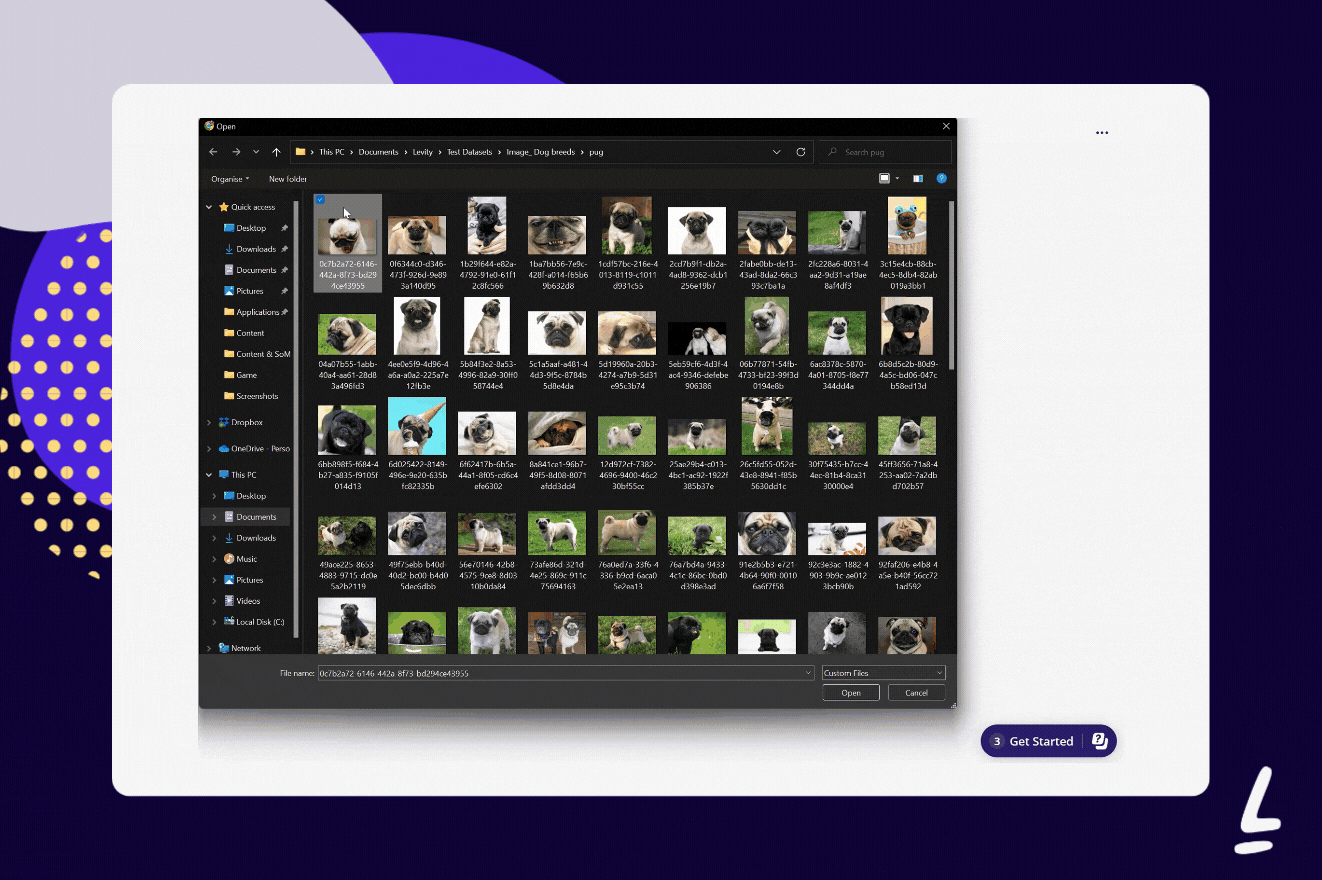
Training your data with an AI tool is as easy as hitting go and waiting for the results. The AI model analyzes your data in order to make accurate predictions on new data—but these predictions are subject to a degree of uncertainty.
You need to then consider the summary, performance score, and suggestions on how to improve your performance. You can choose to keep a Human-in-the-Loop or not. This means that you can keep monitoring the model and its performance by evaluating a percentage of its predictions or leave it to work independently.
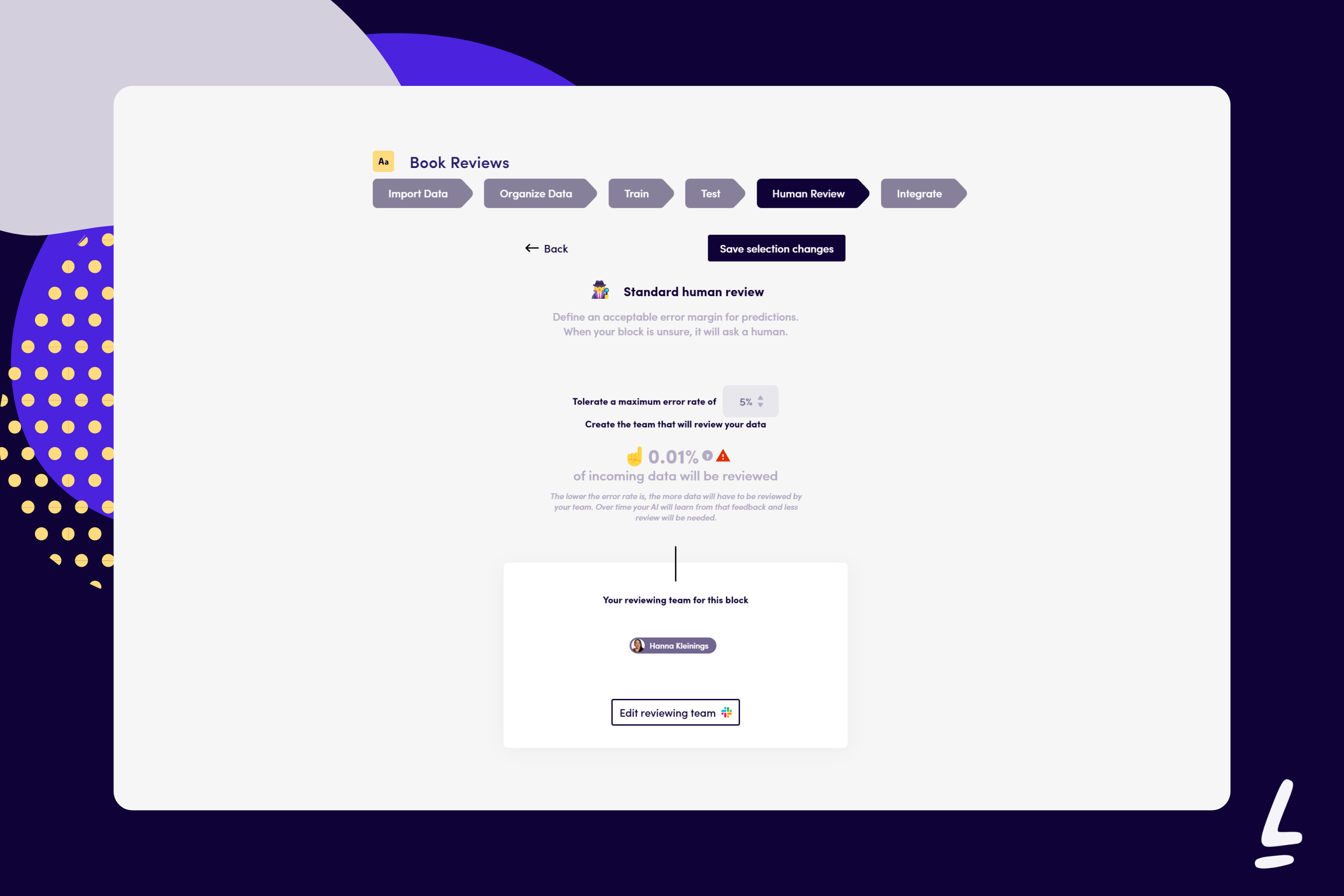
Once you’ve trained the AI model with your data, you’re ready to set up its next steps. Essentially—what should your model do once it’s reached a decision on each piece of data? This is where AI flows come in handy.
Connect to your workflow
Finally, all that’s left is to connect your model to a workflow thanks to the integrations Levity provides. These include Google Sheets, Zapier, Zendesk, Intercom, and many others.
This is the final step of your automation and also the most important one. This is where you define input and output—where the machine gets the data from, and the actions to be taken once the data has been evaluated and categorized.
For example, you can store the output data in a Google Sheet. Or, you can transfer it to another tool connected with Zapier. It all depends on your needs and processes, and your desired use for AI customer support solutions.
Wrapping up on AI for customer support
Using AI for customer support automates many processes and saves you a lot of time and money. You’ll become more efficient and get smarter insights from what your customers are saying.
Increased efficiency and quality of your customer support processes lead to happier customers. They become brand advocates and boost the reputation of your business—good testimonials attract more customers and lead to higher revenues.
By automatically identifying incoming service requests, Levity helps your customer care professionals to spend more time on essential clients. Sign up for Levity today and find out how you could improve your customer support with easy-to-use, no-code AI workflows.
Now that you're here
Levity is a tool that allows you to train AI models on images, documents, and text data. You can rebuild manual workflows and connect everything to your existing systems without writing a single line of code.If you liked this blog post, you'll love Levity.
Sign upNow that you're here
Levity is a tool that allows you to train AI models on images, documents, and text data. You can rebuild manual workflows and connect everything to your existing systems without writing a single line of code.If you liked this blog post, you'll love Levity.
Sign upMore from our Blog
Stay inspired
Sign up and get thoughtfully curated content delivered to your inbox.